Collaborators on the Omdena iRAP 8 Week Challenge which aimed to crowd source AI-based solutions to map crash risk and generate iRAP star rating attribute data have shared some of their publication results.
Earlier this year, 50 AI and machine learning experts including aspiring students, data scientists, ML and data engineers, and domain experts from around the world worked with members of the iRAP Innovation and Development Team focussed on finding a solution for the accelerated and intelligent collection and coding of road attribute data (AiRAP) to prevent road crashes and save lives.
Read these insightful and well applied Python methods:
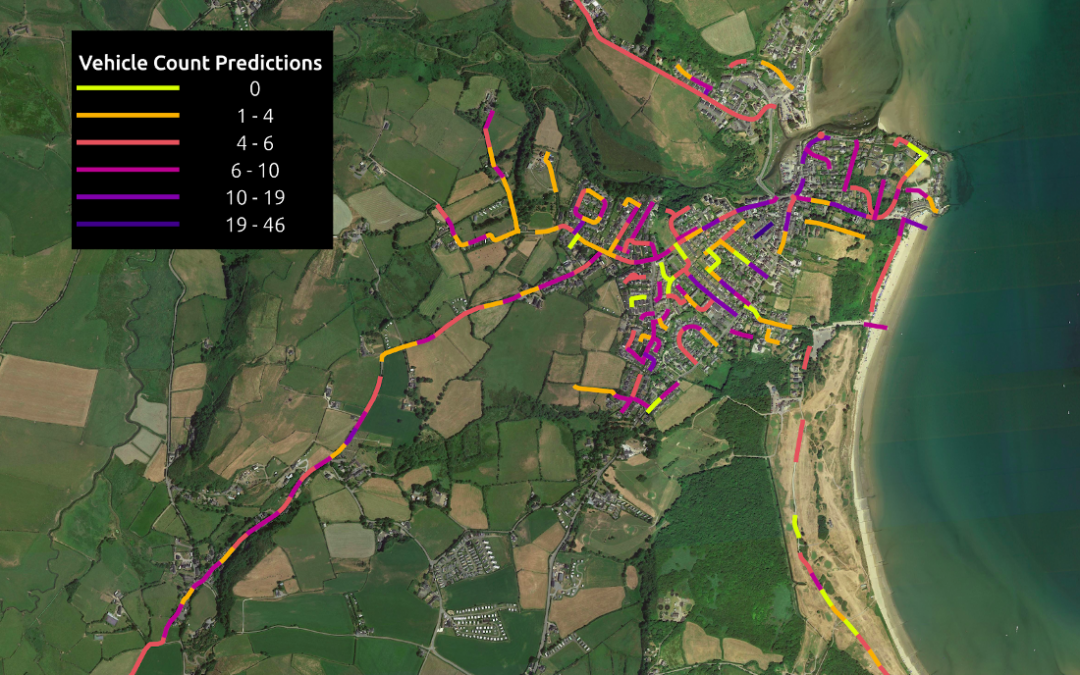
Using Convolutional Neural Networks To Improve Road Safety And Save Lives” by Lois Anne Leal and Chaitree Baradkar – https://bit.ly/3gK8men
“Feature Analysis and Machine Learning for Road Safety” by Leon Hamnett
https://bit.ly/3gJGuqt
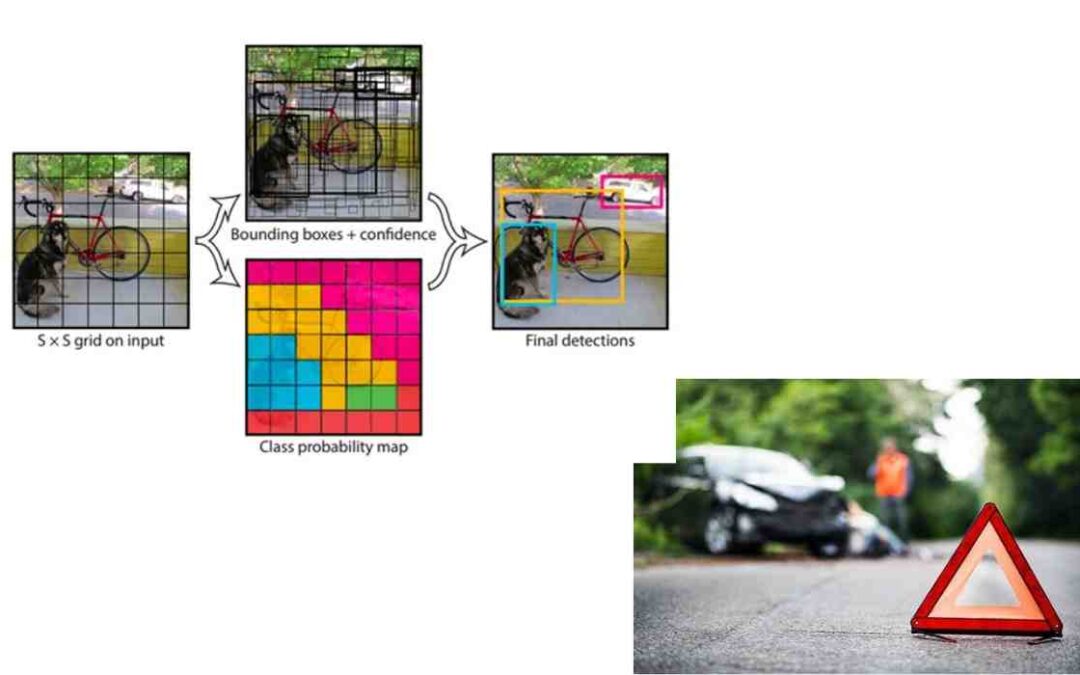
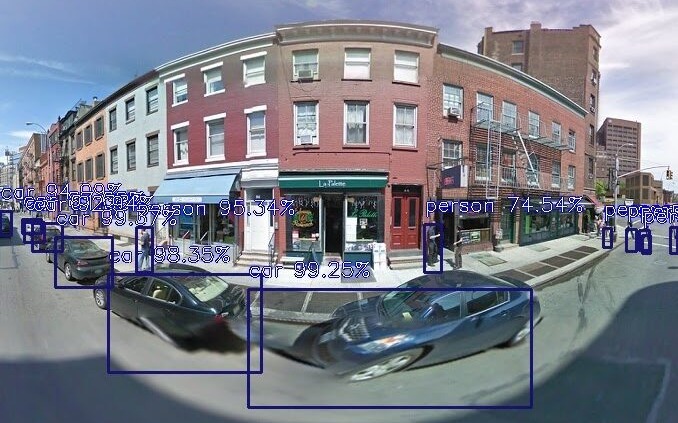
“Object Detection in 2D images to locate cars and pedestrians” by Giulio Giaconi and Dave Bunten
https://bit.ly/3vce0cV
“Advanced EDA of United Kingdom’s Road Safety Data using Python” by Nabanita Roy
https://bit.ly/3dY8l4v
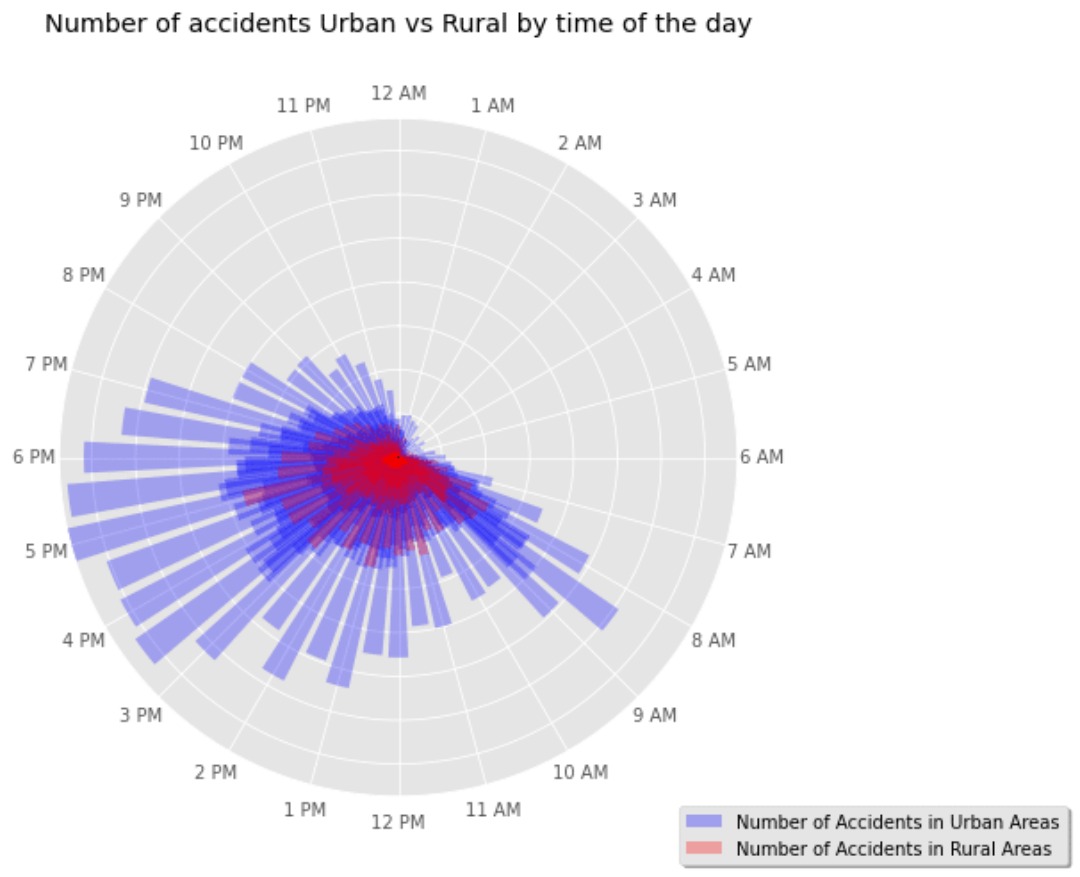
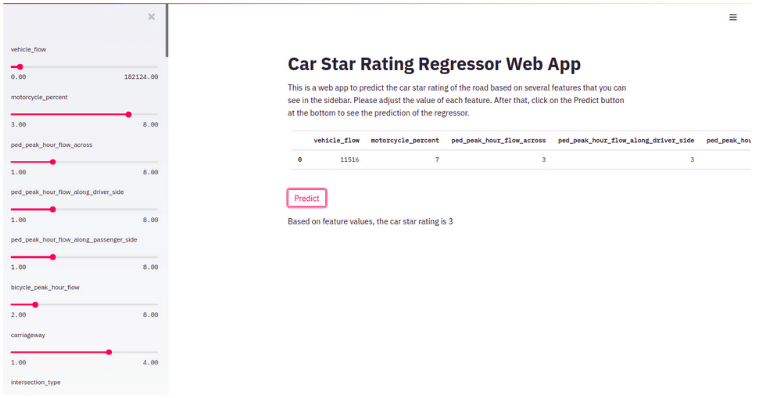
“Streamlit 101: Deploying an AutoML Streamlit Dashboard to Predict and Show Results” by MUHAMMAD ALI
https://bit.ly/3vov9QP
“Learning OpenCV from Scratch to Build a Pedestrian Detector” by Rutuja Kawade
https://bit.ly/2PsvPoS
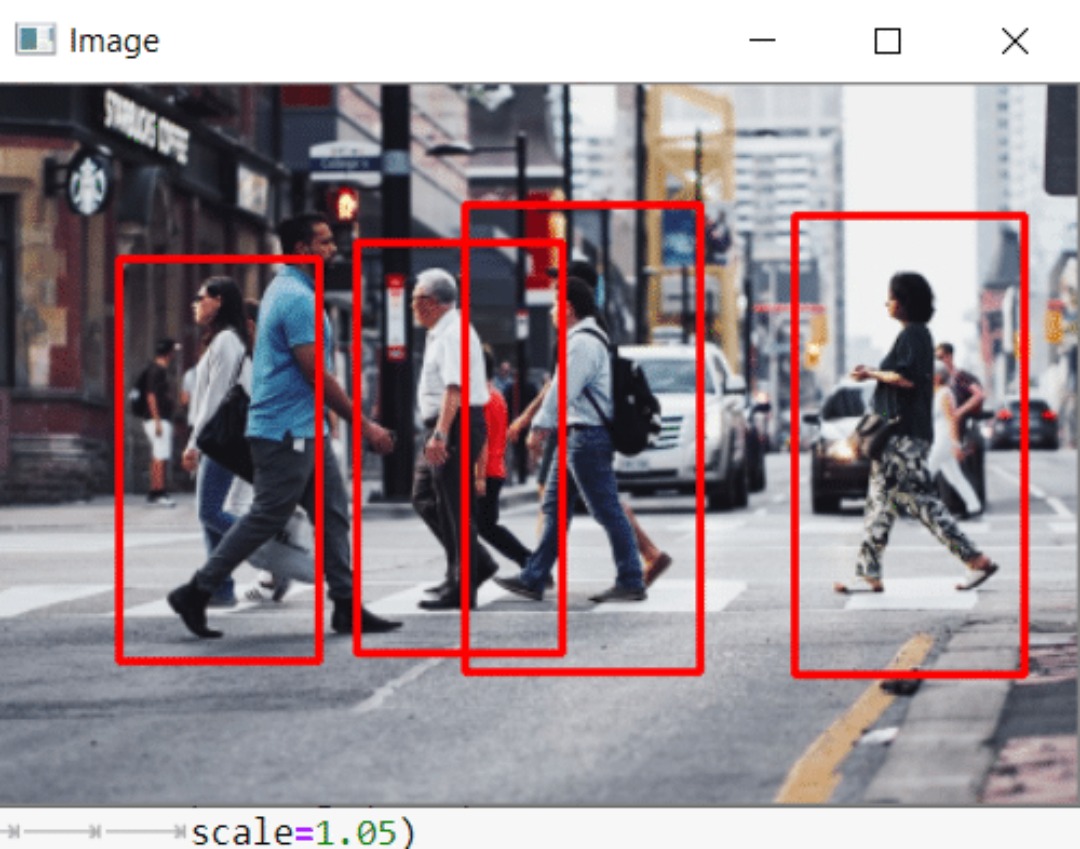
iRAP extends its sincere thanks to the Omdena team. The results will inform big steps forward for #3starorbetter roads for all road users that will save lives.